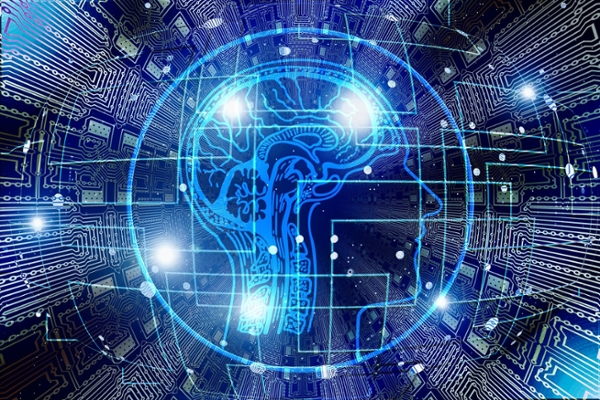
Artificial Intelligence and Decision-Making
Artificial intelligence (AI) presents an interesting set of opportunities and challenges for regulatory systems writ large. AI has a spectrum of possible outcomes. Some people think AI will become the computer that answers all the questions that could ever be asked or go beyond our ability as human beings to compute and choose.
By Peter W.B. Phillips, JSGS Distinguished Professor and CSIP ResearcherArtificial intelligence (AI) presents an interesting set of opportunities and challenges for regulatory systems writ large. AI has a spectrum of possible outcomes. Some people think AI will become the computer that answers all the questions that could ever be asked or go beyond our ability as human beings to compute and choose. While that sounds like an interesting endgame, most of the people who are working on building the algorithms that underlie AI say that they are only going to be an adjunct to decision making and not replace human decision makers. AI will allow for more timely and fulsome engagement with a myriad of data and will present it in ways that will influence decision makers. So, firstly, AI is not going to replace human decision-making systems, especially regulatory systems; but it is going to be part of it. The question then is, what part will AI actually play?
Those who are excited by the prospects of machine learning assisting human decision-making often assert that AI will speed things up and allow us to find nuances and connections that humans would only find after the fact. Getting to this point will require some engagement with both the inside of the algorithm and how the algorithms and their outputs actually get used by humans and human decision-making systems.
Inside the algorithms is an interesting space which is quite transparent at one level. Specialists say AI algorithms are pretty much open source. The things that do the steps that are required to compute the dynamic elements of a dataset are there, but the learning populations are not there and are not public. Algorithms are trained on real or artificial data, but that part is kept secret. So, everybody gets to use the tool, but it is human ingenuity that decides what the learning is anchored on and what reference points we will use. These are important to decision making because you can influence the outcomes of decision rubrics and tools depending on how you define the evidence that you are going to investigate. This part is currently proprietary and is a trade secret.
At the other end of the spectrum is the nature of the multiple iterative computations that have taken these tools and applied them to a learning population to draw inferences and advice out of them. This part is somewhat more transparent, but it is part of the whole system. There is a real question about auditing and accountability. Who decides what or how weights emerge is important because the machines may assign weights that may or may not reflect our preferences and our choices as society.
This is a big part of AI and just another extension of the debate about how evidence should influence public policy. Every piece of evidence at some point or another is subjective, regardless of how objectively we define, describe, measure, or use it. What we choose to make evidence is a preference.
The big challenge is to determine how algorithms can become transparent enough for the regulatory systems to see that they are not manipulating and distorting public interests and intentions. The flip side is that humans will not necessarily make the right choice just because a machine tells you what it thinks is the right answer. We have agency and the ability pick another option in spite of what the machine thinks or says. If anything, AI could tip us to the extremes of decision making, unless we are thoughtful about how we use the data.
AI is here to stay but, like a lot of things, it is oversold and underdeveloped. It will eventually find its home in most human decision systems but will not replace the human being. Like any automated system, it reduces some of the mundane and problematic steps in decision systems and, if properly sited, should provide more autonomy for people to make better decisions with more information, rather than replace us in the decision grid.